Presentations
A journey through unrolling for inverse problems
10 Apr 2024, At CIMS - Imaging inverse problems and generating models -- Edinburgh
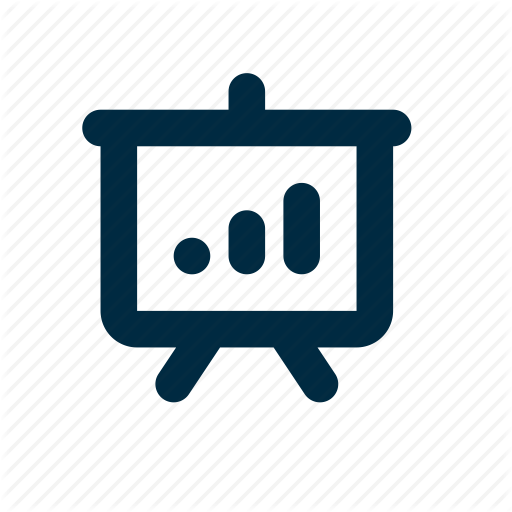
10 Apr 2024, At CIMS - Imaging inverse problems and generating models -- Edinburgh
Inverse problems are ubiquitous in observational science such as imaging, neurosciences or astrophysics. They consist in recovering a signal given noisy observations through a measurement operator. To solve such problems, Machine learning approaches have been proposed based on algorithm unrolling. With such techniques, classical optimization algorithms used to solve inverse problems can be seen as differentiable procedures with parameters that can be learned. In this talk, I will ...
Inverse problems are ubiquitous in observational science such as imaging, neurosciences or astrophysics. They consist in recovering a signal given noisy observations through a measurement operator. To solve such problems, Machine learning approaches have been proposed based on algorithm unrolling. With such techniques, classical optimization algorithms used to solve inverse problems can be seen as differentiable procedures with parameters that can be learned.
In this talk, I will present results that aim at understanding what unrolled algorithms learn and their link to the original associated bilevel problem. Based on results from [Ablin et al 2019], I show that in the smooth case, the gradient of the unrolled problem aligns with the ones of the original problem. Then, I will present contributions from [Malézieux et al. 2022] that show that in the non-smooth case, the jacobian estimation is unstable and too many iterations can be harmful.
Finally, I will present recent results from [Ramzi et al. 2023] showing that when unrolled networks are learned with fixed number of iteration, it is not beneficial to use more iteration at test time.
A framework for bilevel optimization that enables stochastic and global variance reduction algorithms
22 Aug 2023, At ICIAM - Tokyo - Japan
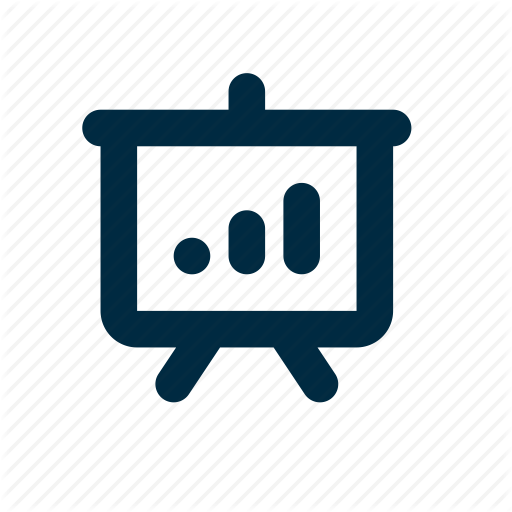
22 Aug 2023, At ICIAM - Tokyo - Japan
Bilevel optimization, the problem of minimizing a value function that involves the arg-minimum of another function, appears in many areas of machine learning. In a large-scale empirical risk minimization setting where the number of samples is huge, it is crucial to develop stochastic methods, which only use a few samples at a time to progress. However, computing the gradient of the value function involves solving a linear system, which makes it difficult to derive unbiased ...
Bilevel optimization, the problem of minimizing a value function that involves the arg-minimum of another function, appears in many areas of machine learning. In a large-scale empirical risk minimization setting where the number of samples is huge, it is crucial to develop stochastic methods, which only use a few samples at a time to progress. However, computing the gradient of the value function involves solving a linear system, which makes it difficult to derive unbiased stochastic estimates. To overcome this problem we introduce a novel framework, in which the solution of the inner problem, the solution of the linear system, and the main variable evolve at the same time. These directions are written as a sum, making it straightforward to derive unbiased estimates. The simplicity of our approach allows us to develop global variance reduction algorithms, where the dynamics of all variables are subject to variance reduction. This allows to design near-optimal algorithms to solve the bi-level problem.
Convolutional Sparse Coding for Electromagnetic Brain Signals
11 Jul 2023, At MLMDA Seminar - Saclay
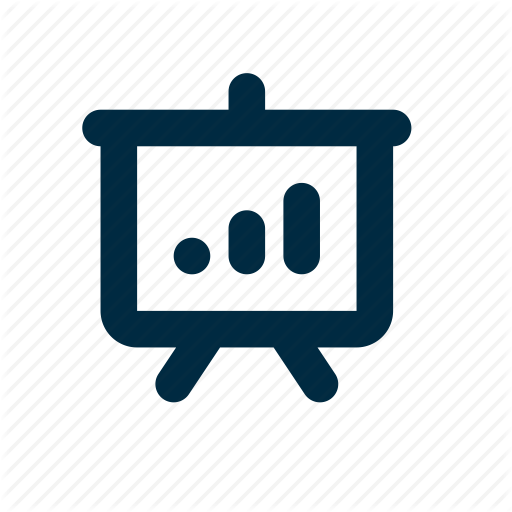
11 Jul 2023, At MLMDA Seminar - Saclay
The quantitative analysis of non-invasive electrophysiology signals from electroencephalography (EEG) and magnetoencephalography (MEG) boils down to the identification of temporal patterns such as evoked responses, transient bursts of neural oscillations but also blinks or heartbeats for data cleaning. An emerging community aims at extracting these patterns efficiently in a non-supervised way, e.g., using Convolutional Dictionary Learning (CDL). Using low rank atoms, mulit-variate CDL is ...
The quantitative analysis of non-invasive electrophysiology signals from electroencephalography (EEG) and magnetoencephalography (MEG) boils down to the identification of temporal patterns such as evoked responses, transient bursts of neural oscillations but also blinks or heartbeats for data cleaning. An emerging community aims at extracting these patterns efficiently in a non-supervised way, e.g., using Convolutional Dictionary Learning (CDL). Using low rank atoms, mulit-variate CDL is able to learn not only prototypical temporal waveforms, but also associated spatial patterns so their origin can be localized in the brain, which leads to an event-based description of the data. Given these events and patterns, a natural question is to estimate how their occurrences are modulated by certain cognitive tasks and experimental manipulations. To address it, we consider a Point-Process (PP) approach. While PP have been used in neuroscience in the past, in particular for single cell recordings (spike trains), techniques such as CDL makes them amenable to human studies based on EEG/MEG signals. We develop a novel statistical PP model – coined driven temporal point processes (DriPP) – where the intensity function of the PP model is linked to a deterministic point process corresponding to stimulation events. Results on MEG datasets demonstrate that our methodology allows to reveal event-related neural responses – both evoked or induced – and isolate non-task specific temporal patterns.
Inverse problems are ubiquitous in observational science such as imaging, neurosciences or astrophysics. They consist in recovering a signal given noisy observations through a measurement operator. To solve such problems, Machine learning approaches have been proposed based on algorithm unrolling. With such techniques, classical optimization algorithms used to solve inverse problems can be seen as differentiable procedure with parameters that can be learned. In this talk, I will ...
Inverse problems are ubiquitous in observational science such as imaging, neurosciences or astrophysics. They consist in recovering a signal given noisy observations through a measurement operator. To solve such problems, Machine learning approaches have been proposed based on algorithm unrolling. With such techniques, classical optimization algorithms used to solve inverse problems can be seen as differentiable procedure with parameters that can be learned.
In this talk, I will focus on the understudied case where no ground truth is available for the problem, i.e., the signal itself can never be observed, only its measurememts. First, I will present results that aim at understanding what unrolled algorithms learn when used to solve an optimization problem. In particular, results from [Ablin et al., 2019, Learning step sizes for unfolded sparse coding] show that learned algorithms cannot go faster than the original algorithm assymptotically, only improve the first iterations. This shows that unrolling should mostly be used with low number of iterations. Then, I will show how algorithm unrolling can be used in conjonction with dictionaries to learn a data-driven structure on the inverse problem solution. For this problem also, we show in [Malézieux et al., 2022, Understanding approximate and unrolled dictionary learning for pattern recovery] that using a few unrolled iterations can provide benefit for learning dictionary while too many iterations can be harmful.
Modeling Brain Waveforms with Convolutional Dictionary Learning and Point Processes
25 Apr 2023, At Ockham seminar, Lyon, France
25 Apr 2023, At Ockham seminar, Lyon, France
The quantitative analysis of non-invasive electrophysiology signals from electroencephalography (EEG) and magnetoencephalography (MEG) boils down to the identification of temporal patterns such as evoked responses, transient bursts of neural oscillations but also blinks or heartbeats for data cleaning. An emerging community aims at extracting these patterns efficiently in a non-supervised way, e.g., using Convolutional Dictionary Learning (CDL). Using low-rank atoms, multivariate CDL is ...
The quantitative analysis of non-invasive electrophysiology signals from electroencephalography (EEG) and magnetoencephalography (MEG) boils down to the identification of temporal patterns such as evoked responses, transient bursts of neural oscillations but also blinks or heartbeats for data cleaning. An emerging community aims at extracting these patterns efficiently in a non-supervised way, e.g., using Convolutional Dictionary Learning (CDL). Using low-rank atoms, multivariate CDL is able to learn not only prototypical temporal waveforms but also associated spatial patterns so their origin can be localized in the brain, which leads to an event-based description of the data. Given these events and patterns, a natural question is to estimate how their occurrences are modulated by certain cognitive tasks and experimental manipulations. To address it, we consider a Point-Process (PP) approach. While PPs have been used in neuroscience in the past, in particular for single-cell recordings (spike trains), techniques such as CDL makes them amenable to human studies based on EEG/MEG signals. We develop a novel statistical PP model – coined driven temporal point processes (DriPP) – where the intensity function of the PP model is linked to a deterministic point process corresponding to stimulation events. Results on MEG datasets demonstrate that our methodology allows revealing event-related neural responses – both evoked or induced – and isolates non-task-specific temporal patterns.
What guarantees for Unrolled Algorithms in Unsupervised Inverse Problems
06 Apr 2023, At MIT, Cambridge, USA
06 Apr 2023, At MIT, Cambridge, USA
Inverse problems are ubiquitous in observational science such as imaging, neurosciences or astrophysics. They consist in recovering a signal given noisy observations through a measurement operator. To solve such problems, Machine learning approaches have been proposed based on algorithm unrolling. With such techniques, classical optimization algorithms used to solve inverse problems can be seen as differentiable procedure with parameters that can be learned.In this talk, ...
Inverse problems are ubiquitous in observational science such as imaging, neurosciences, or astrophysics. They consist in recovering a signal given noisy observations through a measurement operator. To solve such problems, Machine learning approaches have been proposed based on algorithm unrolling. With such techniques, classical optimization algorithms used to solve inverse problems can be seen as differentiable procedures with parameters that can be learned.
In this talk, I will focus on the understudied case where no ground truth is available for the problem, i.e., the signal itself can never be observed, only its degraded measurements. First, I will present results that aim at understanding what unrolled algorithms learn when used to solve an optimization problem. In particular, results from [Ablin et al., 2019, Learning step sizes for unfolded sparse coding] show that learned algorithms cannot go faster than the original algorithm asymptotically, only improving the first iterations. This shows that unrolling should mostly be used with a low number of iterations. Then, I will show how algorithm unrolling can be used in conjunction with dictionaries to learn a data-driven structure on the inverse problem solution. For this problem also, we show in [Malézieux et al., 2022, Understanding approximate and unrolled dictionary learning for pattern recovery] that using a few unrolled iterations can provide benefit for learning dictionary while too many iterations can be harmful.
Benchopt: Reproducible, efficient and collaborative optimization benchmarks
15 Feb 2023, At Reproducible research conference, MVA, ENS Paris-Saclay, France
15 Feb 2023, At Reproducible research conference, MVA, ENS Paris-Saclay, France
Numerical validation is at the core of machine learning research as it allows us to assess the actual impact of new methods and to confirm the agreement between theory and practice. Yet, the rapid development of the field poses several challenges: researchers are confronted with a profusion of methods to compare, limited transparency and consensus on best practices, as well as tedious re-implementation work. As a result, validation is often very partial, which can lead to wrong ...
Numerical validation is at the core of machine learning research as it allows us to assess the actual impact of new methods and to confirm the agreement between theory and practice. Yet, the rapid development of the field poses several challenges: researchers are confronted with a profusion of methods to compare, limited transparency and consensus on best practices, as well as tedious re-implementation work. As a result, validation is often very partial, which can lead to wrong conclusions that slow down the progress of research. In order to solve this, efficient frameworks and tools are required to make the ML benchmarks easily reproducible and extendable. In this talk, I will discuss what are the constraints to producing reproducible research benchmarks. I will also present Benchopt, a collaborative framework to automate, reproduce and publish benchmarks in machine learning, as well as the necessary steps to go from individual research code to reproducible and maintained open-source software.
Convolutional Sparse Coding for Electromagnetic Brain Signals
04 Jul 2022, At AI4SIP - Institut Pascal
04 Jul 2022, At AI4SIP - Institut Pascal
The quantitative analysis of non-invasive electrophysiology signals from electroencephalography (EEG) and magnetoencephalography (MEG) boils down to the identification of temporal patterns such as evoked responses, transient bursts of neural oscillations but also blinks or heartbeats for data cleaning. An emerging community aims at extracting these patterns efficiently in a non-supervised way, e.g., using Convolutional Dictionary Learning (CDL). Using low rank atoms, mulit-variate CDL is ...
The quantitative analysis of non-invasive electrophysiology signals from electroencephalography (EEG) and magnetoencephalography (MEG) boils down to the identification of temporal patterns such as evoked responses, transient bursts of neural oscillations but also blinks or heartbeats for data cleaning. An emerging community aims at extracting these patterns efficiently in a non-supervised way, e.g., using Convolutional Dictionary Learning (CDL). Using low rank atoms, mulit-variate CDL is able to learn not only prototypical temporal waveforms, but also associated spatial patterns so their origin can be localized in the brain, which leads to an event-based description of the data. Given these events and patterns, a natural question is to estimate how their occurrences are modulated by certain cognitive tasks and experimental manipulations. To address it, we consider a Point-Process (PP) approach. While PP have been used in neuroscience in the past, in particular for single cell recordings (spike trains), techniques such as CDL makes them amenable to human studies based on EEG/MEG signals. We develop a novel statistical PP model – coined driven temporal point processes (DriPP) – where the intensity function of the PP model is linked to a deterministic point process corresponding to stimulation events. Results on MEG datasets demonstrate that our methodology allows to reveal event-related neural responses – both evoked or induced – and isolate non-task specific temporal patterns.
SHINE: Sharing the Inverse Estimate for bi-level optimization
20 Jun 2022, At Curves & Surfaces - Arcachon, France
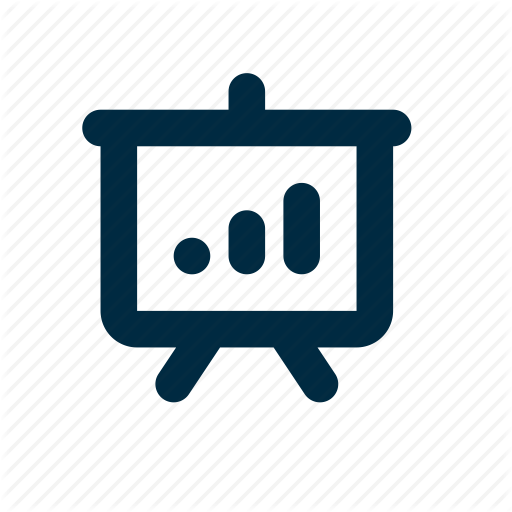
20 Jun 2022, At Curves & Surfaces - Arcachon, France
In recent years, bi-level optimization has raised much interest in the machine learning community, in particular for hyper-parameters optimization and implicit deep learning. This type of problems is often tackled using first-order that requires the computation of the gradient whose expression can be obtained using the implicit function theorem.
In recent years, bi-level optimization has raised much interest in the machine learning community, in particular for hyper-parameters optimization and implicit deep learning. This type of problems is often tackled using first-order that requires the computation of the gradient whose expression can be obtained using the implicit function theorem. The computation of this gradient requires the computation of matrix-vector products involving the inverse of a large matrix which is computationally demanding.
In our work, we propose a novel strategy coined SHINE to tackle this computational bottleneck when the inner problem can be solved with a quasi-Newton algorithm. The main idea is to use the quasi-Newton matrices estimated from the resolution of the inner problem to efficiently approximate the inverse matrix in the direction needed for the gradient computation. We prove that under some restrictive conditions, this strategy gives a consistent estimate of the true gradient.
In addition, by modifying the quasi-Newton updates, we provide theoretical guarantees that our method asymptotically estimates the true implicit gradient under weaker hypothesis.
We empirically study this approach in many settings, ranging from hyperparameter optimization to large Multiscale Deep Equilibrium models applied to CIFAR and ImageNet. We show that it reduces the computational cost of the backward pass by up to two orders of magnitude. All this is achieved while retaining the excellent performance of the original models in hyperparameter optimization and on CIFAR, and giving encouraging and competitive results on ImageNet.
The quantitative analysis of non-invasive electrophysiology signals from electroencephalography (EEG) and magnetoencephalography (MEG) boils down to the identification of temporal patterns such as evoked responses, transient bursts of neural oscillations but also blinks or heartbeats for data cleaning. An emerging community aims at extracting these patterns efficiently in a non-supervised way, e.g., using Convolutional Dictionary Learning (CDL).
The quantitative analysis of non-invasive electrophysiology signals from electroencephalography (EEG) and magnetoencephalography (MEG) boils down to the identification of temporal patterns such as evoked responses, transient bursts of neural oscillations but also blinks or heartbeats for data cleaning. An emerging community aims at extracting these patterns efficiently in a non-supervised way, e.g., using Convolutional Dictionary Learning (CDL). Using low rank atoms, mulit-variate CDL is able to learn not only prototypical temporal waveforms, but also associated spatial patterns so their origin can be localized in the brain, which leads to an event-based description of the data. Given these events and patterns, a natural question is to estimate how their occurrences are modulated by certain cognitive tasks and experimental manipulations. To address it, we consider a Point-Process (PP) approach. While PP have been used in neuroscience in the past, in particular for single cell recordings (spike trains), techniques such as CDL makes them amenable to human studies based on EEG/MEG signals. We develop a novel statistical PP model – coined driven temporal point processes (DriPP) – where the intensity function of the PP model is linked to a deterministic point process corresponding to stimulation events. Results on MEG datasets demonstrate that our methodology allows to reveal event-related neural responses – both evoked or induced – and isolate non-task specific temporal patterns.
In recent years, bi-level optimization -- solving an optimization problem that depends on the results of another optimization problem -- has raised much interest in the machine learning community. A core question for such problem is the estimation of the gradient when the inner problem is not solved exactly. While some fundamental results exist, there is still a gap between what is used in practice and our understanding of the theoretical behavior of such problems.
In recent years, bi-level optimization -- solving an optimization problem that depends on the results of another optimization problem -- has raised much interest in the machine learning community. A core question for such problem is the estimation of the gradient when the inner problem is not solved exactly. While some fundamental results exist, there is still a gap between what is used in practice and our understanding of the theoretical behavior of such problems.
In this talk, I will review different use cases where this type of problem arise, such as hyper-parameter optimization or dictionary learning. I will also review recent advances on how to solve them efficiently.
In recent years, bi-level optimization -- solving an optimization problem that depends on the results of another optimization problem -- has raised much interest in the machine learning community. A core question for such problem is the estimation of the gradient when the inner problem is not solved exactly. While some fundamental results exist, there is still a gap between what is used in practice and our understanding of the theoretical behavior of such problems.
In recent years, bi-level optimization -- solving an optimization problem that depends on the results of another optimization problem -- has raised much interest in the machine learning community. A core question for such problem is the estimation of the gradient when the inner problem is not solved exactly. While some fundamental results exist, there is still a gap between what is used in practice and our understanding of the theoretical behavior of such problems.
In this talk, I will review different use cases where this type of problem arise, such as hyper-parameter optimization or dictionary learning. I will also review recent advances on how to solve them efficiently.
In this talk, I will first review how one can design unrolled algorithms to solve the linear regression with l1 or TV regularization, with a particular focus on the choice of parametrization and loss. Then, I will discuss the reasons why such procedure can lead to better results compared to classical optimization, with a particular focus on the choice of step sizes.
When solving multiple optimization problems sharing the same underlying structure, using iterative algorithms designed for worst case scenario can be considered as inefficient. When one aim at having good solution in average, it is possible to improve the performances by learning the weights of a neural networked designed to mimic an unfolded optimization algorithm. However, the reason why learning the weights of such a network would accelerate the problem resolution is not always clear.
In this talk, I will first review how one can design unrolled algorithms to solve the linear regression with l1 or TV regularization, with a particular focus on the choice of parametrization and loss. Then, I will discuss the reasons why such procedure can lead to better results compared to classical optimization, with a particular focus on the choice of step sizes.
Linked papers:
- Moreau & Bruna. Understanding Neural Sparse Coding with Matrix Factorization. ICLR 2017.
- Ablin, Moreau, Massias & Gramfort. Learning step sizes for unfolded sparse coding. NeurIPS 2019.
- Cherkaoui, Sulam & Moreau. Learning to solve TV regularised problems with unrolled algorithms. NeurIPS 2020.
Learning to optimize with unrolled algorithms
01 Apr 2021, At MOD seminar - University of Tübingen (online)
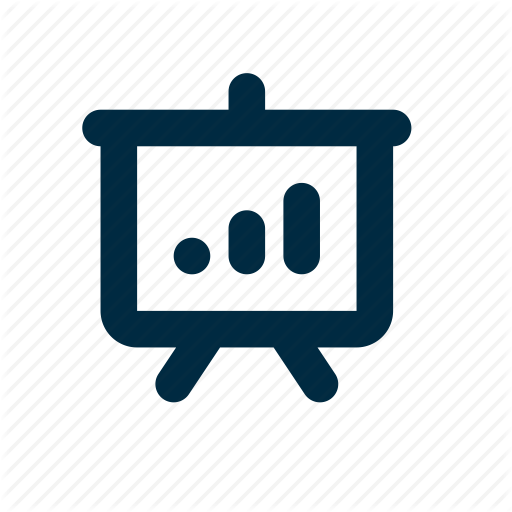
01 Apr 2021, At MOD seminar - University of Tübingen (online)
In this talk, I will first review how one can design unrolled algorithms to solve the linear regression with l1 or TV regularization, with a particular focus on the choice of parametrization and loss. Then, I will discuss the reasons why such procedure can lead to better results compared to classical optimization, with a particular focus on the choice of step sizes.
When solving multiple optimization problems sharing the same underlying structure, using iterative algorithms designed for worst case scenario can be considered as inefficient. When one aim at having good solution in average, it is possible to improve the performances by learning the weights of a neural networked designed to mimic an unfolded optimization algorithm. However, the reason why learning the weights of such a network would accelerate the problem resolution is not always clear.
In this talk, I will first review how one can design unrolled algorithms to solve the linear regression with l1 or TV regularization, with a particular focus on the choice of parametrization and loss. Then, I will discuss the reasons why such procedure can lead to better results compared to classical optimization, with a particular focus on the choice of step sizes.
Linked papers:
- Moreau & Bruna. Understanding Neural Sparse Coding with Matrix Factorization. ICLR 2017.
- Ablin, Moreau, Massias & Gramfort. Learning step sizes for unfolded sparse coding. NeurIPS 2019.
- Cherkaoui, Sulam & Moreau. Learning to solve TV regularised problems with unrolled algorithms. NeurIPS 2020.
Unfolding and learning weights of ISTA using neural networks is a practical way to accelerate the Lasso resolution. However, the reason why learning the weights of such a network would accelerate sparse coding are not clear. In this talk, we look at this problem from the point of view of selecting adapted step sizes for ISTA.
Sparse coding is typically solved by iterative optimization techniques, such as the Iterative Shrinkage-Thresholding Algorithm (ISTA). Unfolding and learning weights of ISTA using neural networks is a practical way to accelerate estimation. However, the reason why learning the weights of such a network would accelerate sparse coding are not clear. In this talk, we look at this problem from the point of view of selecting adapted step sizes for ISTA. We show that a simple step size strategy can improve the convergence rate of ISTA by leveraging the sparsity of the iterates. However, it is impractical in most large-scale applications. Therefore, we propose a network architecture where only the step sizes of ISTA are learned. We demonstrate if the learned algorithm converges to the solution of the Lasso, its last layers correspond to ISTA with learned step sizes. Experiments show that learning step sizes can effectively accelerate the convergence when the solutions are sparse enough.
Multivariate Convolutional Sparse Coding for Electromagnetic Brain Signals
26 Feb 2020, At GT PASADENA
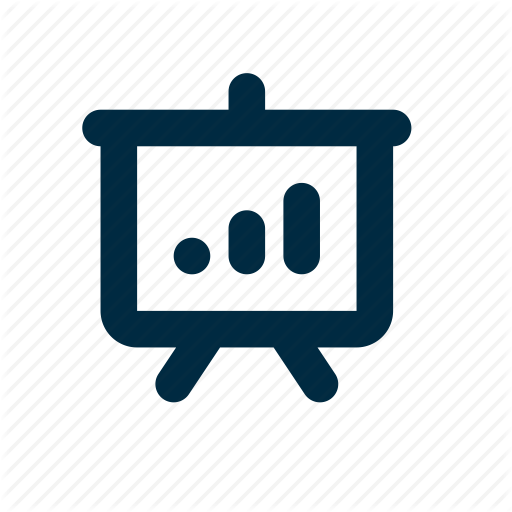
26 Feb 2020, At GT PASADENA
Convolutional dictionary learning algorithm adapted to capture waveforms from MEG signals.
Frequency-specific patterns of neural activity are traditionally interpreted as sustained rhythmic oscillations, and related to cognitive mechanisms such as attention, high level visual processing or motor control. While alpha waves (8--12\, Hz) are known to closely resemble short sinusoids, and thus are revealed by Fourier analysis or wavelet transforms, there is an evolving debate that electromagnetic neural signals are composed of more complex waveforms that cannot be analyzed by linear filters and traditional signal representations. In this paper, we propose to learn dedicated representations of such recordings using a multivariate convolutional sparse coding (CSC) algorithm. Applied to electroencephalography (EEG) or magnetoencephalography (MEG) data, this method is able to learn not only prototypical temporal waveforms, but also associated spatial patterns so their origin can be localized in the brain. Our algorithm is based on alternated minimization and a greedy coordinate descent solver that leads to state-of-the-art running time on long time series. To demonstrate the implications of this method, we apply it to MEG data and show that it is able to recover biological artifacts. More remarkably, our approach also reveals the presence of non-sinusoidal mu-shaped patterns, along with their topographic maps related to the somatosensory cortex.
Unfolding and learning weights of ISTA using neural networks is a practical way to accelerate the Lasso resolution. However, the reason why learning the weights of such a network would accelerate sparse coding are not clear. In this talk, we look at this problem from the point of view of selecting adapted step sizes for ISTA.
Sparse coding is typically solved by iterative optimization techniques, such as the Iterative Shrinkage-Thresholding Algorithm (ISTA). Unfolding and learning weights of ISTA using neural networks is a practical way to accelerate estimation. However, the reason why learning the weights of such a network would accelerate sparse coding are not clear. In this talk, we look at this problem from the point of view of selecting adapted step sizes for ISTA. We show that a simple step size strategy can improve the convergence rate of ISTA by leveraging the sparsity of the iterates. However, it is impractical in most large-scale applications. Therefore, we propose a network architecture where only the step sizes of ISTA are learned. We demonstrate if the learned algorithm converges to the solution of the Lasso, its last layers correspond to ISTA with learned step sizes. Experiments show that learning step sizes can effectively accelerate the convergence when the solutions are sparse enough.
In this talk, we show that adaptive analytic step sizes can be used to improved ISTA. This steps can also be learned using a neural network architecture simlar to LISTA, which is theoretically the only way to learn to accelerate ISTA asymptotically.
Sparse coding is typically solved by iterative optimization techniques, such as the Iterative Shrinkage-Thresholding Algorithm (ISTA). Unfolding and learning weights of ISTA using neural networks is a practical way to accelerate estimation. In this paper, we study the selection of adapted step sizes for ISTA. We show that a simple step size strategy can improve the convergence rate of ISTA by leveraging the sparsity of the iterates. However, it is impractical in most large-scale applications. Therefore, we propose a network architecture where only the step sizes of ISTA are learned. We demonstrate that for a large class of unfolded algorithms, if the algorithm converges to the solution of the Lasso, its last layers correspond to ISTA with learned step sizes. Experiments show that our method is competitive with state-of-the-art networks when the solutions are sparse enough.
Best Practices & Pitfalls in Applying Machine Learning to Magnetic Resonance Imaging
11 May 2019, At Invited talk @ ISMRM - Montreal, Canada
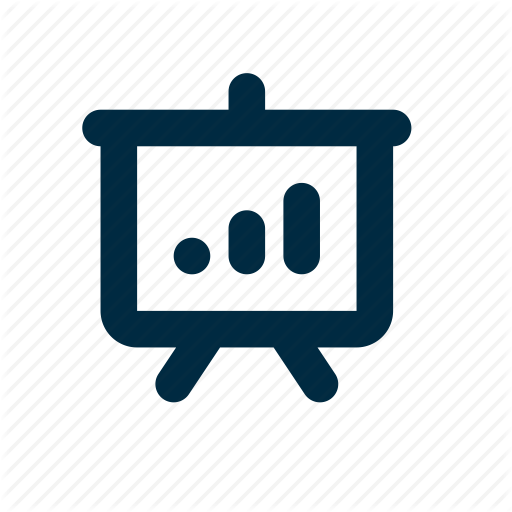
11 May 2019, At Invited talk @ ISMRM - Montreal, Canada
In this talk, I cover the concept of generalization for supervised learning, with a focus on model selection and the importance of sample size.
In this talk, I cover the concept of generalization for supervised learning, with a focus on model selection and the importance of sample size.
Distributed Convolutional Dictionary Learning (DiCoDiLe): Pattern Discovery in Large Images and Signals
16 Apr 2019, At Parietal seminar - INRIA, Saclay
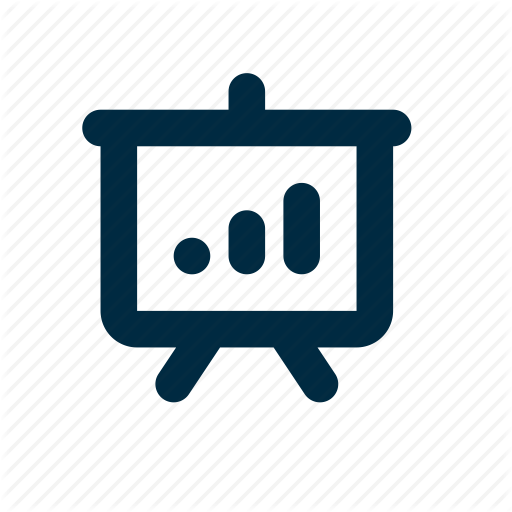
16 Apr 2019, At Parietal seminar - INRIA, Saclay
DiCoDiLe: a distributed and asynchronous algorithm, employing locally greedy coordinate descent and an asynchronous locking mechanism that does not require a central server.
Convolutional dictionary learning (CDL) estimates shift invariant basis adapted to multidimensional data. CDL has proven useful for image denoising or inpainting, as well as for pattern discovery on multivariate signals. As estimated patterns can be positioned anywhere in signals or images, optimization techniques face the difficulty of working in extremely high dimensions with millions of pixels or time samples, contrarily to standard patch-based dictionary learning. To address this optimization problem, this work proposes a distributed and asynchronous algorithm, employing locally greedy coordinate descent and an asynchronous locking mechanism that does not require a central server. This algorithm can be used to distribute the computation on a number of workers which scales linearly with the encoded signal's size. Experiments confirm the scaling properties which allows us to learn patterns on large scales images from the Hubble Space Telescope.
Multivariate Convolutional Sparse Coding for Electromagnetic Brain Signals
28 Mar 2019, At Séminaire SMILE - Paris
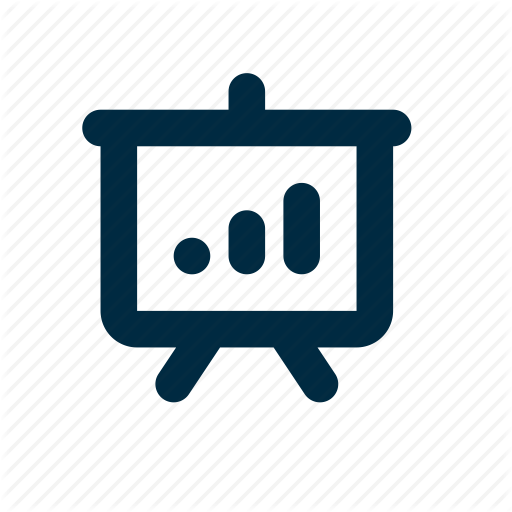
28 Mar 2019, At Séminaire SMILE - Paris
Convolutional dictionary learning algorithm adapted to capture waveforms from MEG signals.
Frequency-specific patterns of neural activity are traditionally interpreted as sustained rhythmic oscillations, and related to cognitive mechanisms such as attention, high level visual processing or motor control. While alpha waves (8--12\, Hz) are known to closely resemble short sinusoids, and thus are revealed by Fourier analysis or wavelet transforms, there is an evolving debate that electromagnetic neural signals are composed of more complex waveforms that cannot be analyzed by linear filters and traditional signal representations. In this paper, we propose to learn dedicated representations of such recordings using a multivariate convolutional sparse coding (CSC) algorithm. Applied to electroencephalography (EEG) or magnetoencephalography (MEG) data, this method is able to learn not only prototypical temporal waveforms, but also associated spatial patterns so their origin can be localized in the brain. Our algorithm is based on alternated minimization and a greedy coordinate descent solver that leads to state-of-the-art running time on long time series. To demonstrate the implications of this method, we apply it to MEG data and show that it is able to recover biological artifacts. More remarkably, our approach also reveals the presence of non-sinusoidal mu-shaped patterns, along with their topographic maps related to the somatosensory cortex.
Multivariate Convolutional Sparse Coding for Electromagnetic Brain Signals
19 Feb 2019, At MLMDA seminar - CMLA, ENS Paris-Saclay
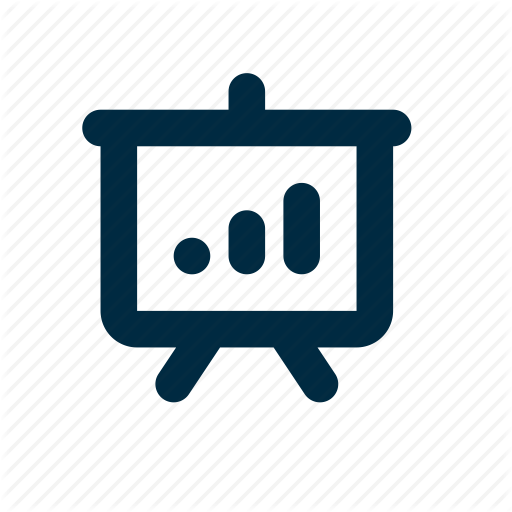
19 Feb 2019, At MLMDA seminar - CMLA, ENS Paris-Saclay
Rank-1 constrined Convolutional Dictionary Learning algorithm for neural time series and distributed algorithm for CDL (DiCoDiLe)
Frequency-specific patterns of neural activity are traditionally interpreted as sustained rhythmic oscillations, and related to cognitive mechanisms such as attention, high level visual processing or motor control. While alpha waves (8--12\,Hz) are known to closely resemble short sinusoids, and thus are revealed by Fourier analysis or wavelet transforms, there is an evolving debate that electromagnetic neural signals are composed of more complex waveforms that cannot be analyzed by linear filters and traditional signal representations. In this paper, we propose to learn dedicated representations of such recordings using a multivariate convolutional sparse coding (CSC) algorithm. Applied to electroencephalography (EEG) or magnetoencephalography (MEG) data, this method is able to learn not only prototypical temporal waveforms, but also associated spatial patterns so their origin can be localized in the brain. Our algorithm is based on alternated minimization and a greedy coordinate descent solver that leads to state-of-the-art running time on long time series. To demonstrate the implications of this method, we apply it to MEG data and show that it is able to recover biological artifacts. More remarkably, our approach also reveals the presence of non-sinusoidal mu-shaped patterns, along with their topographic maps related to the somatosensory cortex.
In a second part, I will present my recent work on a distributed algorithm for CDL, called DiCoDiLe.
Using the Dictionary Structure for efficient Convolutional Dictionary Learning
31 Jan 2019, At Seminaire du LTCI - Télécom paritech
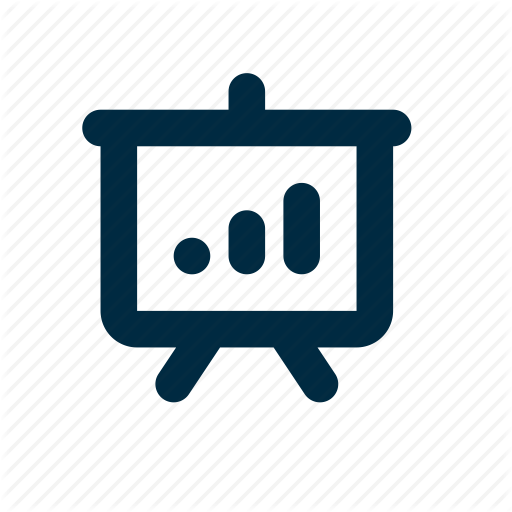
31 Jan 2019, At Seminaire du LTCI - Télécom paritech
Convolutional Dictionary Learning (CDL) is a popular tool in image processing for denoising or inpainting. This technique extends dictionary learning to learn adapted basis that are shift invariant. It can also be used in the context of large multivariate signals to learn and localize recurring patterns. In this talk, I will focus on how the structure of the dictionary can be leveraged to design efficient CDL algorithm and to improve the interpretability of the recovered patterns in MEG signals.
Convolutional Dictionary Learning (CDL) is a popular tool in image processing for denoising or inpainting. This technique extends dictionary learning to learn adapted basis that are shift invariant. It can also be used in the context of large multivariate signals to learn and localize recurring patterns. In this talk, I will focus on how the structure of the dictionary can be leveraged to design efficient CDL algorithm and to improve the interpretability of the recovered patterns in MEG signals.
Gregor and Le Cun (2010) have shown empirically it is possible to estimate the solution of the sparse coding with a neural network called LISTA trained with back-propagation. In the first part of this talk, I will show the link between the performance of this network and the particular structure of the dictionary and in particular the Gram matrix of the problem. This structure is shown to be sufficient to explain the performance of LISTA and numerical experiments show it is also necessary. (Joint work with J. Bruna).
Then, I will focus on the convolutional sparse coding (CSC). The particular properties of the band-circulant matrices allow to derive an efficient algorithm, called Locally Greedy Coordinate Descent (LGCD), to solve the CSC. This algorithm is very efficient in this context as it prioritizes important updates, still managing low iteration complexity. This algorithm can also be used in an asynchronous and distributed setting. We show that even though the updates are only performed locally, without global communication, this distributed algorithm converges to the optimal solution of the CSC. Numerical experiments show that this algorithm has better performance than state-of-the-art algorithms for CSC and that it accelerates super linearly with the number of cores used. (Joint work with L. Oudre, N. Vayatis and A. Gramfort).
In the final part of my talk, I will introduce a novel rank 1 constraint for the learned atoms for practical problems in neuroscience. This constraint, inspired from the underlying physical model for neurological signals, is then used to highlight relevant structure in MEG signals. (Join work with T. Dupré la Tour, M. Jas and A. Gramfort).
Learning Recurring Patterns in Large Signals with Convolutional Dictionary Learning
24 Jan 2019, At Seminar day - CosmoStat, CEA, Orsay
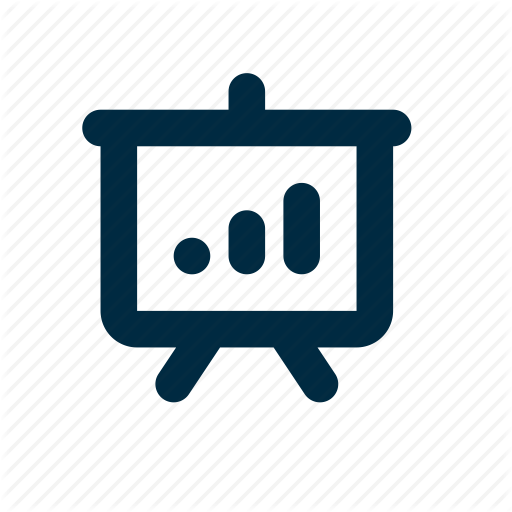
24 Jan 2019, At Seminar day - CosmoStat, CEA, Orsay
How convolutional dictionary learning can be used in the context of large multivariate signals to learn and localize recurring patterns: modelisation and computational aspects.
Convolutional dictionary learning has become a popular tool in image processing for denoising or inpainting. This technique extends dictionary learning to learn adapted basis that are shift invariant. This talk will discuss how this technique can also be used in the context of large multivariate signals to learn and localize recurring patterns. I will discuss both computational aspects, with efficient iterative and distributed convolutional sparse coding algorithms, as well as a novel rank 1 constraint for the learned atoms. This constraint, inspired from the underlying physical model for neurological signals, is then used to highlight relevant structure in MEG signals.
DICOD: Distributed Convolutional Coordinate Descent for Convolutional Sparse Coding
12 Jul 2018, At ICML - Stockholm, Sweden
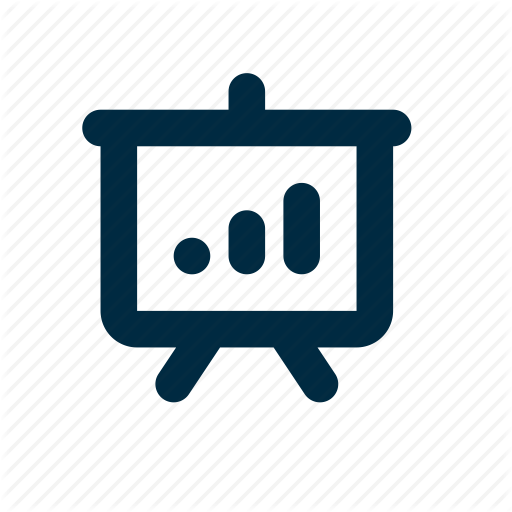
12 Jul 2018, At ICML - Stockholm, Sweden
In this talk, we introduce DICOD, a distributed convolutional sparse coding algorithm to build shift invariant representations for long signals
In this talk, we introduce DICOD, a distributed convolutional sparse coding algorithm to build shift invariant representations for long signals. This algorithm is designed to run in a distributed setting, with local message passing, making it communication efficient. It is based on coordinate descent and uses locally greedy updates which accelerate the resolution compared to greedy coordinate selection. We prove the convergence of this algorithm and highlight its computational speed-up which is super-linear in the number of cores used. We also provide empirical evidence for the acceleration properties of our algorithm compared to state-of-the-art methods.
Multivariate Convolutional Sparse Coding for Electromagnetic Brain Signals
29 May 2018, At Parietal seminar - INRIA, Saclay
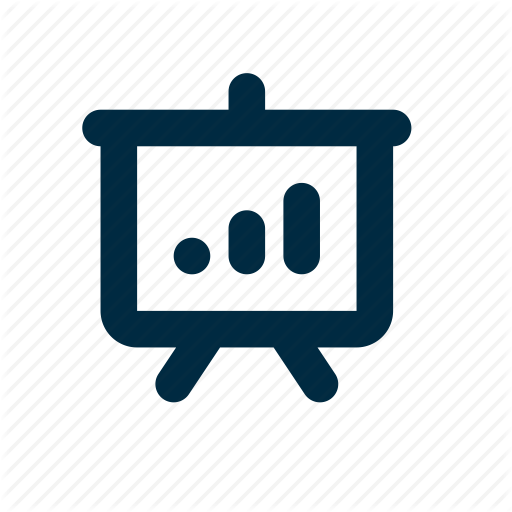
29 May 2018, At Parietal seminar - INRIA, Saclay
A multivariate CSC with rank-1 constrain algorithm designed to study brain activity waveforms
Frequency-specific patterns of neural activity are traditionally interpreted as sustained rhythmic oscillations, and related to cognitive mechanisms such as attention, high level visual processing or motor control. While alpha waves (8-12 Hz) are known to closely resemble short sinusoids, and thus are revealed by Fourier analysis or wavelet transforms, there is an evolving debate that electromagnetic neural signals are composed of more complex waveforms that cannot be analyzed by linear filters and traditional signal representations. In this paper, we propose to learn dedicated representations of such recordings using a multivariate convolutional sparse coding (CSC) algorithm. Applied to electroencephalography (EEG) or magnetoencephalography (MEG) data, this method is able to learn not only prototypical temporal waveforms, but also associated spatial patterns so their origin can be localized in the brain. Our algorithm is based on alternated minimization and a greedy coordinate descent solver that leads to state-of-the-art running time on long time series. To demonstrate the implications of this method, we apply it to MEG data and show that it is able to recover biological artifacts. More remarkably, our approach also reveals the presence of non-sinusoidal mu-shaped patterns, along with their topographic maps related to the somatosensory cortex.
Convolutional Sparse Representations -- application to physiological signals and interpretability for Deep Learning
Convolutional representations extract recurrent patterns which lead to the discovery of local structures in a set of signals. They are well suited to analyze physiological signals which requires interpretable representations in order to understand the relevant information. Moreover, these representations can be linked to deep learning models, as a way to bring interpretability in their internal representations. In this dissertation, we describe recent advances on both computational and theoretical aspects of these models.
Our main contribution in the first part is an asynchronous algorithm, called DICOD, based on greedy coordinate descent, to solve convolutional sparse coding for long signals. Our algorithm has super-linear acceleration. We also explored the relationship of Singular Spectrum Analysis with convolutional representations, as an initialization step for convolutional dictionary learning.
In a second part, we focus on the link between representations and neural networks. Our main result is a study of the mechanisms which accelerate sparse coding algorithms with neural networks. We show that it is linked to a factorization of the Gram matrix of the dictionary. Other aspects of representations in neural networks are also investigated with an extra training step for deep learning, called post-training, to boost the performances of trained networks by improving their last layer's weights.
Finally, we illustrate the relevance of convolutional representations for physiological signals. Convolutional dictionary learning is used to summarize signals from human walking and Singular Spectrum Analysis is used to remove the gaze movement in young infant's oculometric recordings.
Our main contribution in the first part is an asynchronous algorithm, called DICOD, based on greedy coordinate descent, to solve convolutional sparse coding for long signals. Our algorithm has super-linear acceleration. We also explored the relationship of Singular Spectrum Analysis with convolutional representations, as an initialization step for convolutional dictionary learning.
In a second part, we focus on the link between representations and neural networks. Our main result is a study of the mechanisms which accelerate sparse coding algorithms with neural networks. We show that it is linked to a factorization of the Gram matrix of the dictionary. Other aspects of representations in neural networks are also investigated with an extra training step for deep learning, called post-training, to boost the performances of trained networks by improving their last layer's weights.
Finally, we illustrate the relevance of convolutional representations for physiological signals. Convolutional dictionary learning is used to summarize signals from human walking and Singular Spectrum Analysis is used to remove the gaze movement in young infant's oculometric recordings.
Acceleration strategies for sparse coding resolution, using the optmization structure.
Sparse coding is a core building block in many data analysis and machine learning pipelines. Finding good algorithms to accelerate the resolution of such problem is thus critical to many applications.
The first part of this talk is focused on recent acceleration techniques which estimate the sparse code with a train neural network such as LISTA. Empirical results have shown that they achieve high quality estimates with few iterations by modifying the parameters of the proximal splitting appropriately. In this talk, I will link the performance of these network to a factorization of the Gram matrix of the problem which preserves the l1 norm. This mechanism is shown to be sufficient to explain the performance of LISTA and numerical experiments show that it is also necessary. (Joint work with J. Bruna) In a second part of the talk, I will focus on convolutional sparse coding, with band circulant matrices. The particular properties of these problems allow to derive an efficient distributed algorithm based on the greedy coordinate descent. It can be shown that this algorithm converges in an asynchronous setting, is communication efficient and has a super-linear speed-up. These different properties are then illustrated with numerical experiments. (Joint work with N. Vayatis and L. Oudre)
The first part of this talk is focused on recent acceleration techniques which estimate the sparse code with a train neural network such as LISTA. Empirical results have shown that they achieve high quality estimates with few iterations by modifying the parameters of the proximal splitting appropriately. In this talk, I will link the performance of these network to a factorization of the Gram matrix of the problem which preserves the l1 norm. This mechanism is shown to be sufficient to explain the performance of LISTA and numerical experiments show that it is also necessary. (Joint work with J. Bruna) In a second part of the talk, I will focus on convolutional sparse coding, with band circulant matrices. The particular properties of these problems allow to derive an efficient distributed algorithm based on the greedy coordinate descent. It can be shown that this algorithm converges in an asynchronous setting, is communication efficient and has a super-linear speed-up. These different properties are then illustrated with numerical experiments. (Joint work with N. Vayatis and L. Oudre)
Understanding Trainable Sparse Coding with Matrix Factorization
06 Nov 2017, At Tech talk - Google, Zurich
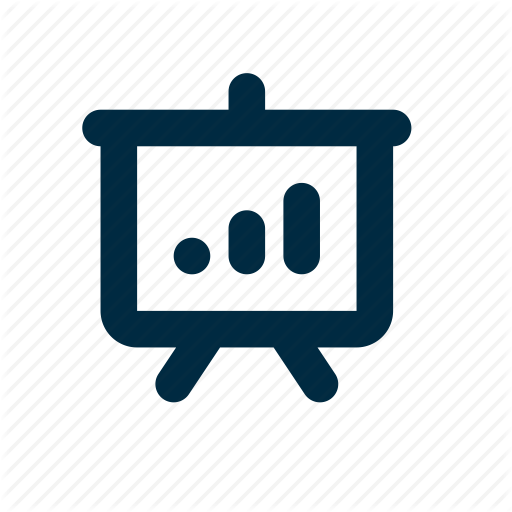
06 Nov 2017, At Tech talk - Google, Zurich
In this talk, we provide elements explaining why Learned ISTA is able to accelerate the LASSO resolution. This vision of optimization algorithms in the neural network framework could be used to link sparse representations and neural networks.
Optimization algorithms for sparse coding can be viewed in the light of the neural network framework. Using this framework, it is possible to design trainable networks which accelerate the resolution of an optimization problem on a given distribution, as it has been shown with the Learned ISTA network, proposed by Gregor & Le Cun (2010).
In this talk, we provide elements explaining why the acceleration is possible in the case of ISTA. We show that the resolution of sparse coding can be accelerated compared to ISTA when the design matrix admits a quasi-diagonal factorization with sparse eigenspaces. The resulting algorithm has the same convergence rate but an improved constant factor. Then we show under which conditions such factorization is possible with high probability for generic Gaussian dictionaries. Finally, we design neural networks which compute this algorithm and show that they are a re-parametrization of LISTA. Thus, the performance of LISTA are at least as good as this algorithm. We conclude by designing adverse examples for our factorization based algorithm and show that LISTA also fails to accelerate on these cases, proving that this mechanism plays a role in LISTA acceleration.
Understanding physiological signals via sparse representations
09 Oct 2017, At Journée de rentré EDMH - IHES, Orsay
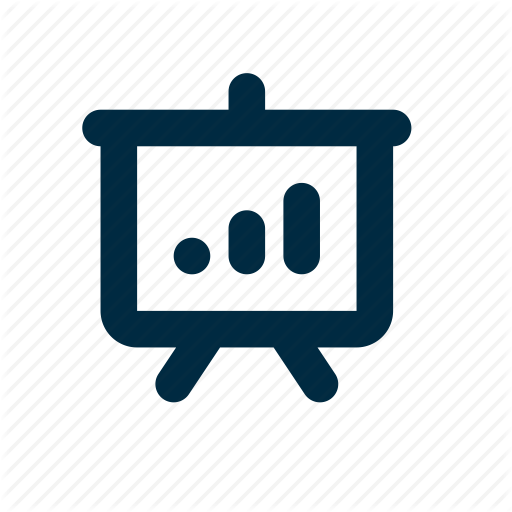
09 Oct 2017, At Journée de rentré EDMH - IHES, Orsay
General presentation of time series representations and of the convolutional representation model.
General presentation of time series representations and of the convolutional representation model.
The concurrent.futures module offers an easy to use API to parallelize code execution in python, using threading or multiprocessing primitives. We will begin our talk by presenting this API and the differences between Thread and Process.
The concurrent.futures module offers an easy to use API to parallelize code execution in python, using threading or multiprocessing primitives. We will begin our talk by presenting this API and the differences between Thread and Process.
For Process backed executions, useful for pure python parallelization, several issues can reduce the performance. Spawning new workers for each execution can create a large overhead, but maintaining the pool of worker across the program can become quickly bothersome. We will describe several of the pitfall that can make using concurrent.futures unstable.
Finally, we will introduce loky, a library providing a robust, reusable pool of workers, handled internally. It uses a customized implementation of ProcessPoolExecutor from concurrent.futures. We will describe its main features and the major technical design choice that helped making it more robust.